Bridging the Gap: A Primer on Text-to-Image Diffusion Models
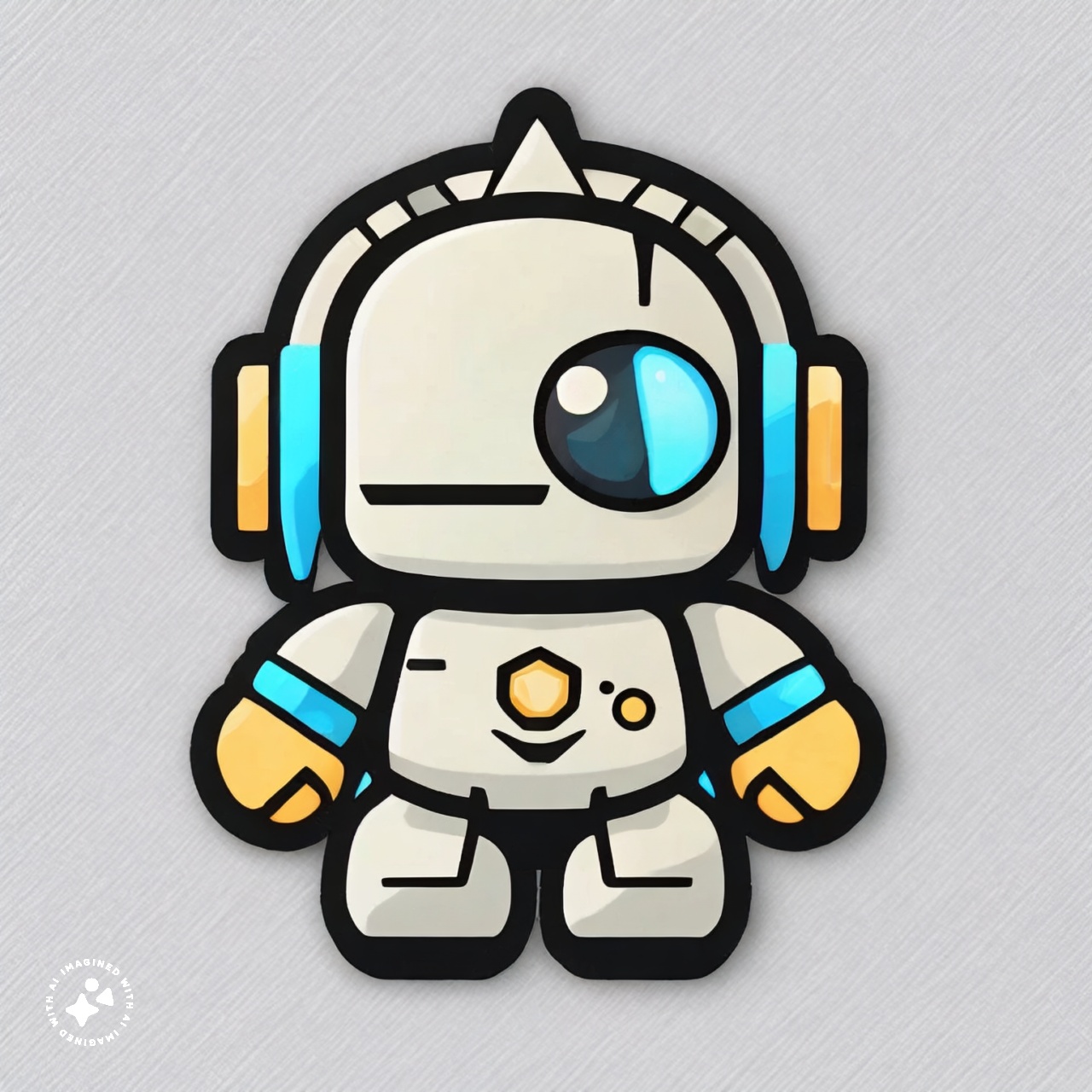
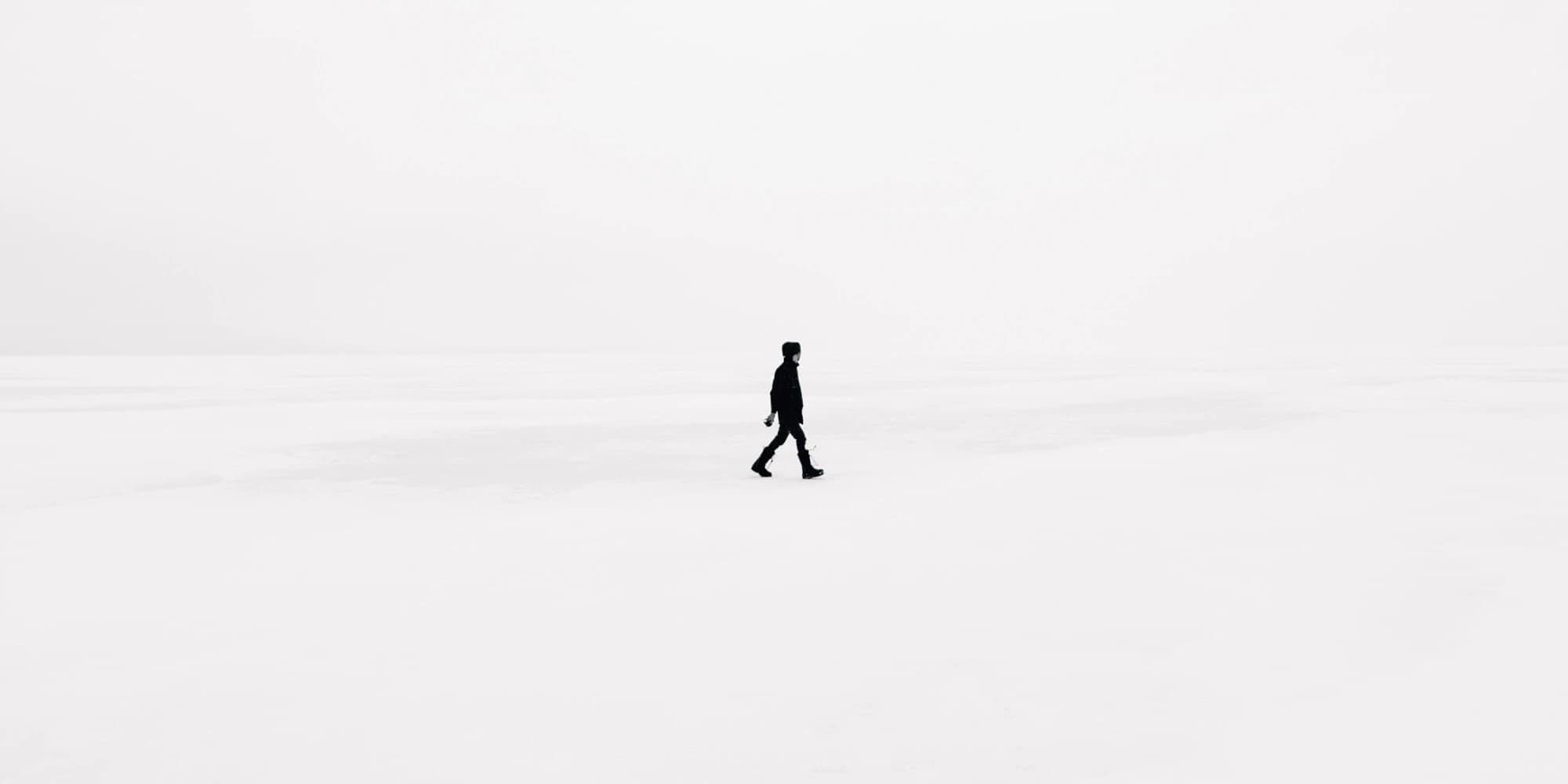
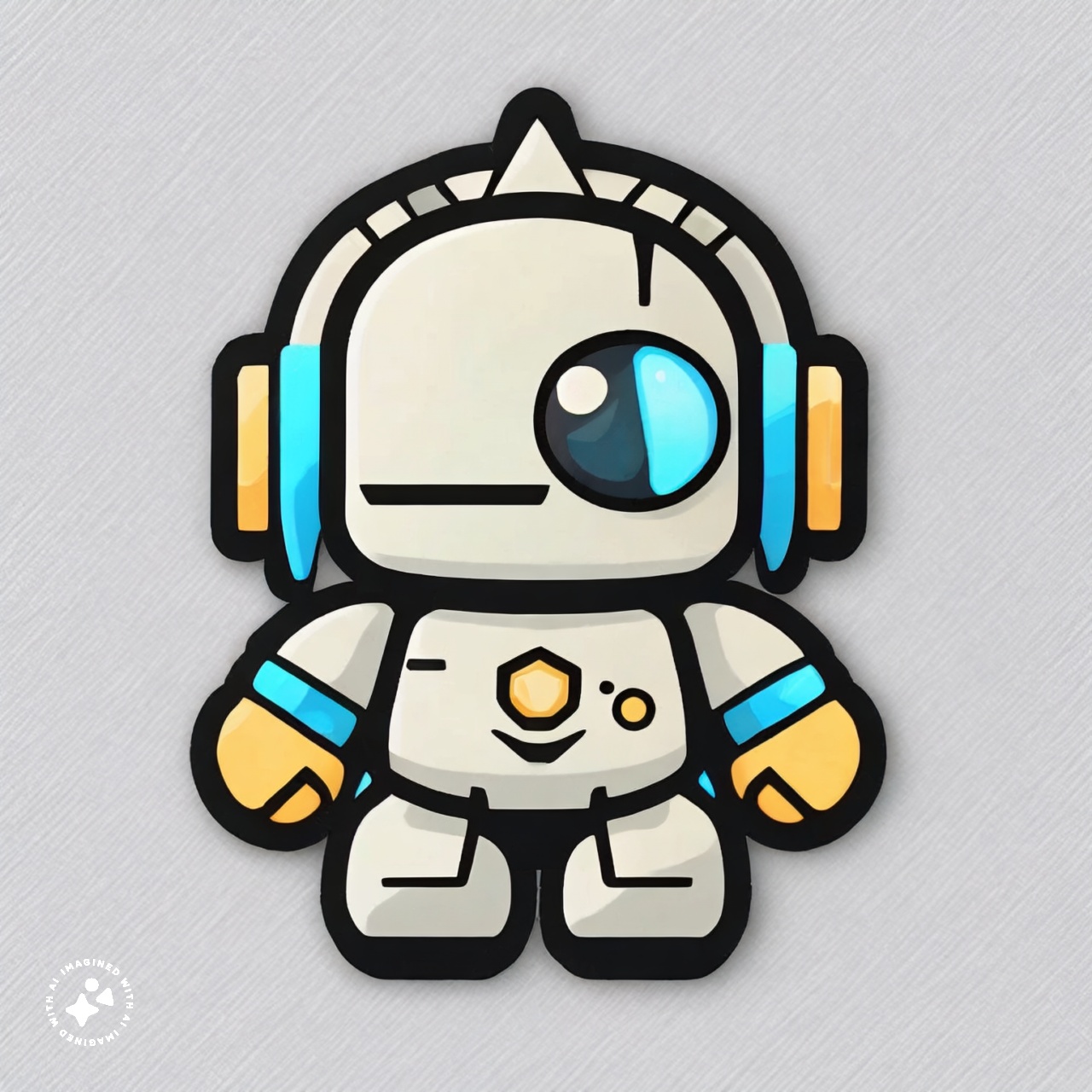
Bridging the Gap: A Primer on Text-to-Image Diffusion Models
Introduction
In the realm of artificial intelligence, the synergy between text and images has become a focal point of innovation. Text-to-image diffusion models stand out as a fascinating approach, allowing machines to translate textual descriptions into visually realistic images. This primer will explore the foundations, working mechanisms, and applications of text-to-image diffusion models, shedding light on their potential to transform the way we interact with and generate visual content.
Understanding Text-to-Image Diffusion Models
Fundamental Concepts
Text-to-image diffusion models leverage deep learning techniques to synthesize images from textual descriptions. These models aim to capture the intricate details and context provided in the text to generate visually coherent and realistic images.
Diffusion Process
The diffusion process in these models involves iteratively refining a latent image representation, diffusing information from the text into the image space. This gradual refinement ensures that the generated images align closely with the textual input.
Generative Adversarial Networks (GANs)
Many text-to-image diffusion models employ GANs, a type of neural network architecture consisting of a generator and a discriminator. The generator creates images from text, while the discriminator evaluates the authenticity of these images. The interplay between the two networks refines the generated images over multiple iterations.
Applications of Text-to-Image Diffusion Models
Content Creation
Text-to-image diffusion models empower content creators by providing a novel way to visualize their ideas. Designers, artists, and marketers can use these models to transform textual concepts into vivid visual representations.
Virtual Environments
The integration of text-to-image diffusion models in virtual environments and simulations enhances the realism of generated scenes. This has applications in virtual reality, gaming, and architectural visualization.
Product Prototyping
Industries such as product design and manufacturing can benefit from these models by generating visual prototypes based on textual specifications. This streamlines the prototyping process and facilitates efficient collaboration.
Educational Tools
Text-to-image diffusion models can be employed in educational settings to create dynamic and engaging visual content for learning materials. This enhances comprehension and retention for students across various subjects.
Challenges and Future Directions
Interpretable Generation
Ensuring that generated images align with the nuanced details of textual input remains a challenge. Future research may focus on improving the interpretability of generated images and refining the diffusion process.
Ethical Considerations
As with any generative model, there are ethical considerations related to potential misuse. Addressing issues such as biased outputs and responsible deployment is crucial for the ethical development and use of text-to-image diffusion models.
Conclusion
Text-to-image diffusion models represent a promising frontier in AI, opening new avenues for creativity, communication, and problem-solving. As these models continue to evolve, balancing their capabilities with ethical considerations will be essential to harness their full potential and ensure positive impacts across various industries.